Paper-cardboard sorting
The industrial pilot at Stora Enso's Langerbrugge Mill
The story
Today, the recycling market is changing rapidly due to global changes where the quality requirements of the incoming and outgoing material are increased. As a result, systems that are separating waste material from the target material need to be improved continuously to cope with this change.
Stora Enso's Langerbrugge Mill in northwest Belgium, which is one of the largest paper mills in Europe, required a more effective paper-cardboard sorting solution and technology that can easily be retrained for anomaly detection. This technology was developed by Robovision, a company specializing in deep learning-based machine vision and robot programming, and Imec, which is the world-leading R&D and innovation hub in nanoelectronics and digital technologies within the framework of the AUTOWARE project.
The Challenge
A big challenge in paper recycling is the separation of cardboard and waste materials from paper. Sometimes, small pieces of cardboard can not be sorted out of the waste paper and cause dirt spots and visible brown fibres in the paper. At the same time glue and plastic lead to black spots and stickies that cause paper breaks.
The quality of the supply stream varies significantly between suppliers and this is becoming a major challenge in recent years. This is mainly because of the increasing number of carton types and several innovations in carton materials themselves as it is becoming increasingly harder to distinguish carton from paper.
Paper/carton come from random sources and have extremely irregular colours, shapes, folds, sizes and densities. Each variable in the input requires additional layers of sorting systems and affects the quality of the output. In contrast to traditional waste separation techniques used in PET or metal recycling such as gravity, magnetic and eddy current separation, paper and carton from uncontrolled sources are difficult to sort. Paper, residue cardboards, greasy or food cartons and plastics need to be removed as they affect the recycling quality.
To solve this challenge in paper recycling plants, it is not enough just to improve the system to decrease the percentage of rest material. The system also needs to evolve from a static system to a dynamic system which can rapidly reconfigure to handle different input batches of quality.
In the current system, mixed paper and carton sources are moved on a conveyor towards an air separator. The air separator separates the paper and carton into two conveyors. One belt recycles the high concentration carton and the other, the high concentration paper. However, on each belt, residual paper or carton still exists and this has to be further separated. Residual carton mixed in paper results in poor quality (black spots) while paper mixed in carton creates a lower grade residual stream.
A major bottleneck in advanced vision systems using deep learning architectures is the initial set-up cost of the system. For a specific task at hand, data needs to be collected beforehand to tune the system which is time-intensive and introduces downtime in the workflow. This time-cost can be split into two relevant parts.
One part is the generation of sufficient so-called ‘training’ examples which the system uses to complete the learning process. The other part is the computational set-up cost of the system. Given the large variance in the paper-cardboard supply, fast retraining and continuous learning strategies are essential to reduce turn-over downtime. For this, the learning models need to efficiently adapt to the new forms of paper and carton in the supply.
The solution
Within the AUTOWARE project, Imec and Robovision created a detection framework which can swiftly change between the materials as well as to train as fast as possible to quickly adapt for different types of cardboard.
For high accuracy and fast detection sorting through camera systems, Robovision has provided and refined a deep learning vision component that is capable to leverage machine learning techniques to enhance vision systems for robots. Robovision has configured deep learning systems and associated tools to create novel machine vision systems.
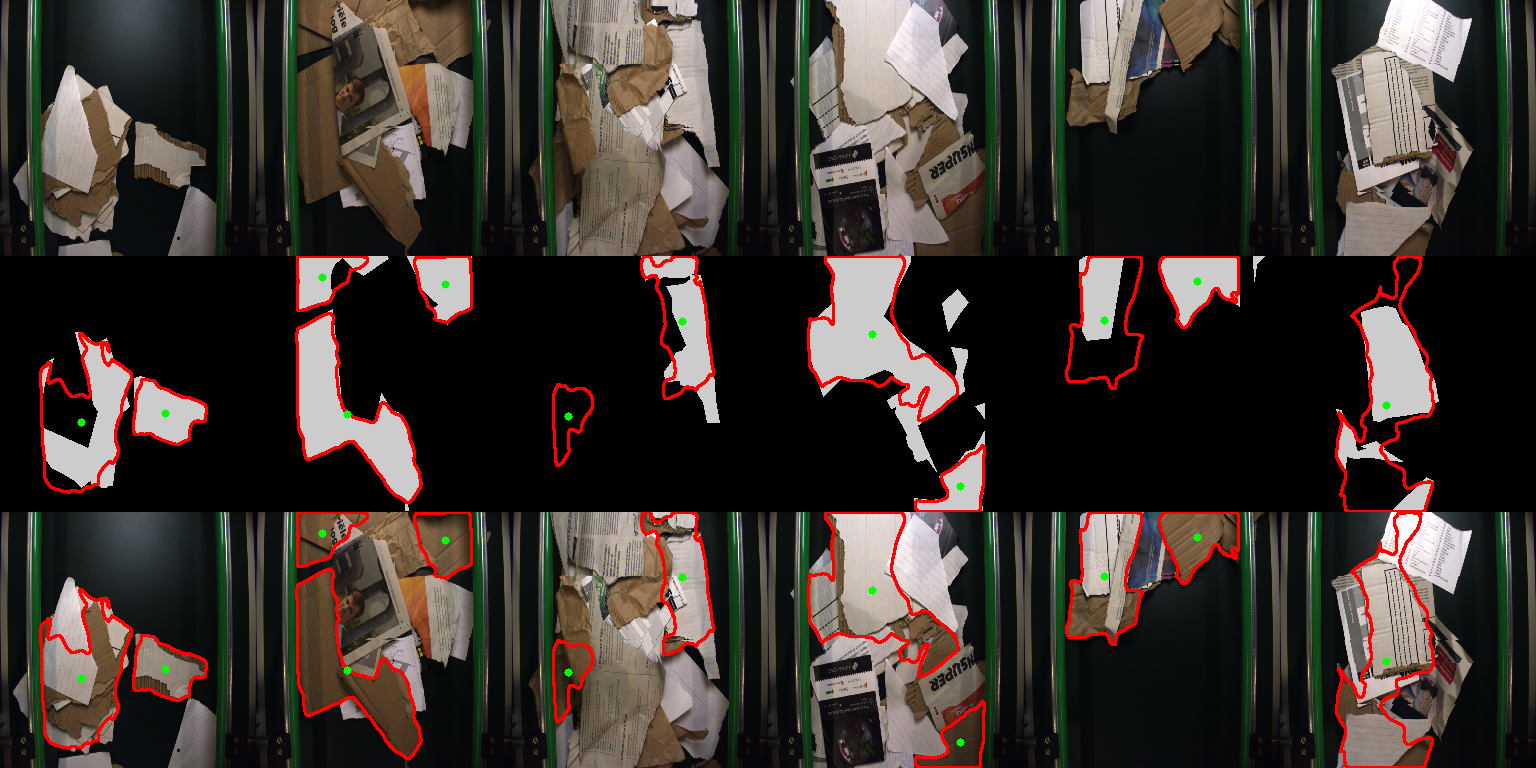
This novel technology offers the possibility to use vision-based algorithms based on combinations of complex features such as shape, form, colour and can be reconfigured to recognize new products, as it was demonstrated in Stora Enso’s Langerbrugge Mill use case.
The adoption of deep learning vision systems effectively removes the need for manual feature engineering, however, it introduces the need to tune the deep neural network to the specific problem. This tuning process requires specialist knowledge. In addition, during set-up, it may require extensive computing power in the form of specific hardware (GPU, TPU). Both problems hinder the adoption of such systems in a manufacturing setting where rapid changeover times are critical.
Imec has developed a specific software to mitigate these problems. GpFlowOpt is a novel and unique library for Bayesian optimization, which can be viewed as a modern spin-off of the widely used SUMO-toolbox. Imec’s software GpFlowOpt addresses the problem of the high computational cost of training deep learning architectures and targets to drastically reduce the training time while achieving better or equivalent performance.
GpFlowOpt also allows the user to speed up expensive simulations and reduce turnover times through the rapid reconfiguration of deep learning vision systems. This software has been adopted to allow more accurate paper-cardboard separation and more efficient tuning of deep learning vision technology.
The results
Machine learning systems and in particular deep learning-based vision technology is evolving rapidly and technologies will need to be swapped out continuously, as a result, the creation and implementation of the reference architecture AUTOWARE is providing aid to keep the system manageable. In addition, these technologies are new and high-risk and the piloting of such an architecture will help Stora Enso and SMEs to evaluate the ROI.
The developed technologies also enable the user to quickly change the type of problem they need to solve. The Stora Enso use case has demonstrated that swift reconfiguration of model parameters is possible. This way, a manufacturer can easily introduce new types of products and get better results using the developed transfer learning and quick reconfiguration tooling.
Key factors for manufacturing SMEs are: agile automation, continuity, accuracy and scalability. The developed AI software platform offers a solution for all these factors. The agile automation is addressed by easily retrainable AI models without the need for rare data scientist profiles and huge investments. This allows SMEs to adapt their production process according to specific requirements of their customers. As a result, SMEs can benefit from the reduced stops in the stream and increased quality of the products.